Acclaimed science fiction writer, Issac Asimov, wrote in his book, I, Robot, “Every period of human development has had its own particular type of human conflict—its own variety of problem.” In our times, it is artificial intelligence. Generative AI created enormous buzz as ChatGPT entered the scene a year back. Its ability to churn out academic essays, compose persuasive business proposals and love letters with similar finesse, and whip up clever ideas brought it instant fame. For a hot moment, it seemed like the shortcut to all life’s problem.
But as uptake increased, disturbing reports started to emerge. Deep flaws were found in the outputs. What seemed a perfect invention became the source of wide-spread anxiety. Of the things written about it, bias and hallucination took centerstage.
In the Ignite Tech Talk recorded at the recent Edge Field Day event, Gina Rosenthal, long-time Field Day panelist and Founder and CEO of Digital Sunshine, picked apart the problems and explained what could go wrong with generative AI tools like ChatGPT.
Volatility of Generative AI Tools
Recently, the capabilities of generative AI came under scrutiny. Two lawyers were fined for acting in bad faith when they submitted a legal research work which turned out to be pure work of fiction. The lawyers took the help of ChatGPT to write the legal briefs, but never fact-checked the inputs. Shockingly, ChatGPT had cooked up false cases to support their argument. The inaccuracies were mind-boggling and left the court baffled and bewildered.
So can generative AI machines be trained to perform tasks reliably? The answer is no, says Gina. That’s because GPT models were originally created to give applications the ability to create human-like text and content. “It’s trained on a bunch of words, and it just does next word prediction,” she says.
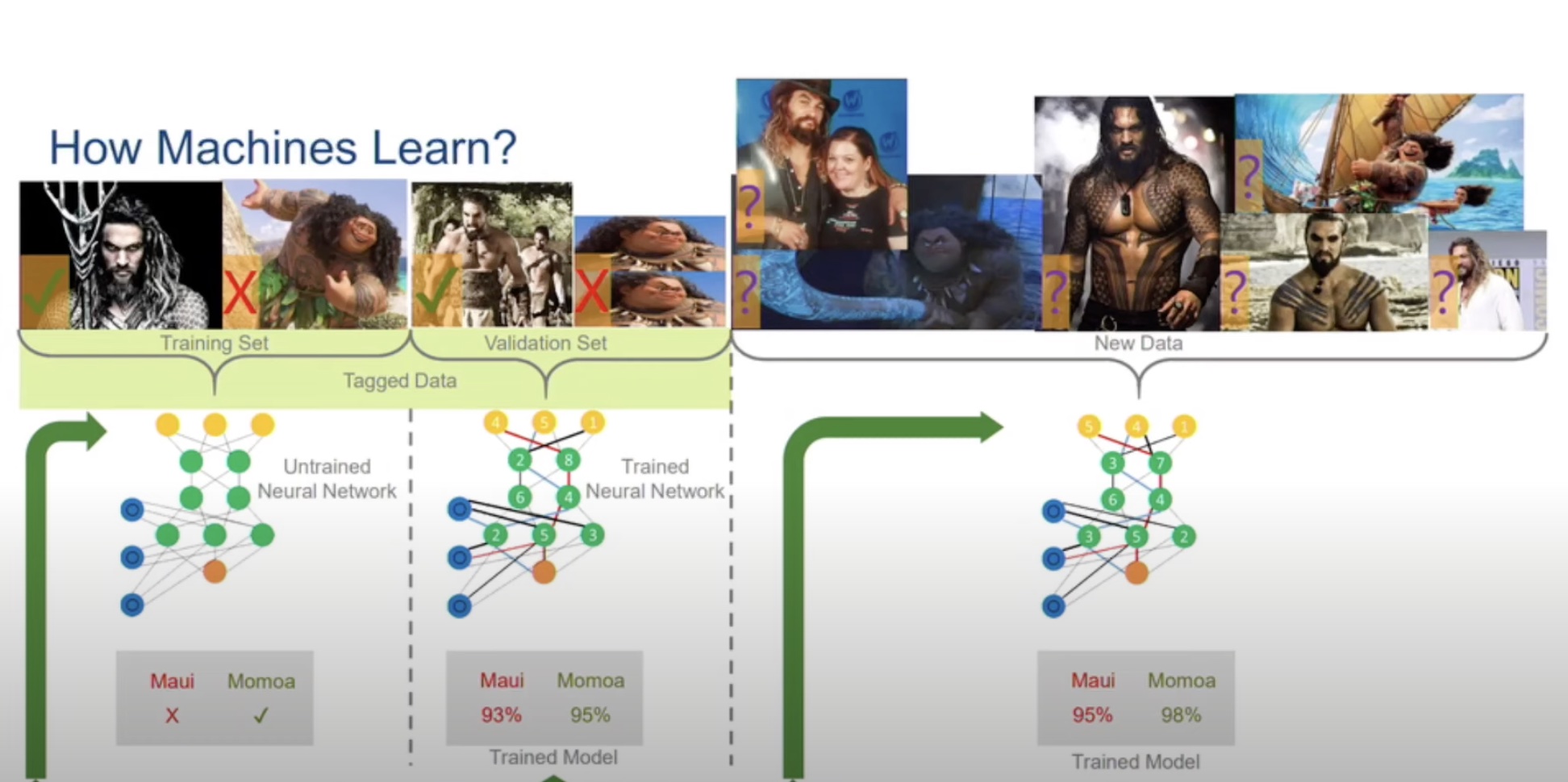
Here’s how the models learn to do the things they do. The way the models are able to distinguish between two objects and classify them correctly is by ingesting and processing a lot of data about them. The neural network which is the AI mechanism that teaches machines to process data similar to how the human brain does, looks at this data. As it processes the data, it learns about the different attributes which make the objects different from each other.
Parameters and weights are used to do high and low-level tuning to maximize the learning rate and quality. The goal is to get the percentage closer to 100%. In the next iterations, fresh data is brought in and the learning keeps on going.
“So it can give you information based on how it was fine-tuned and how those weights were set in the algorithm,” says Rosenthal.
We’ve seen in the past year that AI tools like ChatGPT can pass the bar exam, write scripts and solve problems, and it has made us confident in their abilities. But Rosenthal reminds the audience that it was never meant to do all that. These tools are designed to do much simpler things like auto-complete emails and so on, and if used at scale for complex things, a whole lot can go wrong.
There is robust evidence that generative AI demonstrates strong racial, gender and political biases. When asked a series of questions in a test, it exhibited bias against marginalized people that is likely to amplify discrimination against certain communities.
In hindsight, this was coming a long time. The data used to train GPT models come from all over the Internet. These archives are populated by us humans who have inherently strong biases.
“Like it or not, most of voices on the Internet for the time it has been around have been men, and there’s a lot of bias built in that way,” says Rosenthal. The models absorb all these biases lurking in the data, and encode them in the analysis. As a result, the output is rife with things gender stereotypes, and misclassifications.
“Generative AI does not let out any gender differences or nuances that we’re now learning to appreciate and acknowledge in the world,” she emphasized.
Course Correction
Regardless, generative AI is on the up and up. As Bill Gates famously declared, “The age of AI has begun”, and generative AI heralds application of AI in everyday lives. Experts opine that in the observable future, it will take over the Internet, substituting content creators by a sweeping majority.
The hype is backed by impressive numbers. In just a year, generative AI has become bigger than anyone had ever imagined. According to reports, in 2020, the global generative AI market was valued at $10.14 billion. By 2030, it is predicted to grow at a compound annual growth rate (CAGR) of 35.6%.
If it is going to assume the predicted scale, it is in our interest to perfect the technology the best we can. “We must use humans to clean the data from the dark web atrocities,” says Rosenthal.
In Kenya, workers are paid $1.46 to $3.74 hourly after taxes, to label 70 text passages per nine hour shift. But that has a human cost. “To go into a country and take their best and brightest to train to find the worst things in the web so we don’t have to deal with them is really awful,” she opines.
Environmental Impacts
While efforts are being made to remove biases from the data, it can never be fully possible to do so as long as these models rely on humans to train them.
This problem is intensified by the models’ ability to hallucinate as is evidenced in several cases. People have asked chatbots to book them Airbnbs for their vacations, and have found on arrival that the places don’t exist.
These tools may be highly intelligent, but they are perfectly capable of producing wrong outputs.
In addition to be volatile and alarmingly inaccurate on occasions, Rosenthal pointed out that generative AI also has significant environmental footprints.
“It’s extremely environmentally sensitive to train these algorithms,” she says.
The Co2 emissions for training GPT models are through the roof. Data shows that the emissions are five times that of air travel. In the mid-west, datacenters have caused temperature to soar in the summer, bacause of high emissions.
“We are going to pay environment costs for this. How many diff types of models can we afford to have from an environmental standpoint?” she asks.
Wrapping Up
Playing fast and loose with a technology as powerful as generative AI can have staggering consequences. Leveraging its full potential is not to trust it to make decisions for us or our businesses. We’re not there yet, where we can offload the work entirely to a software, and cut humans out of the equation.
As Rosenthal insists, to set the expectations straight, users must begin by seeing generative AI for what it is. “It’s just a stochastic parrot – it can repeat what it’s told. You can’t teach it to write, be creative or bring something new that humans do. And that is still very powerful. It can enhance your resume or get your research started, but it’s definitely not a substitute for creating your own content, or marketing programs for your company.”
Even if users decide to use these tools heavily in their day to day lives, there needs to be some form of human supervision and guardrails to make sure that they do not derail from their original objectives. For this, humans need to adopt the roles of gatekeepers to elevate the accuracy of information. To that end, policymakers must create and implement regulations to check the ills and impacts.
Meanwhile, to wrap your head around generative AI’s social implications, Rosenthal suggests reading resources on Algorithmic Justice League that spreads awareness on AI bias and helps leverage the technology safely. Be sure to watch the talk – Generative AI Tools, What Could Go Wrong? – and other interesting Ignite Tech Field Day Talks from the recent Edge Field Day event.