Innovation is in full swing in AI. There are big transformations afoot and marvelous things are about to happen. Aside from the metaverse and AI supercomputers, the field of AI/ML is also at the cusp of an explosion of architectural diversity. And the transition from research labs to the marketplace is happening at a fast-track. So that all this does not end up being confounding for new users, we need good benchmarks in machine learning and MLCommons is dedicated to that cause.
MLCommons, an Open Engineering Community
MLCommons measures performances of ML models based on chosen indicators. The test results reveal raw performance numbers of systems, components and configurations that help readers judge the suitability of one system over other for a chosen application. Based on that, enterprises can make purchasing decisions, build new systems, improve existing systems or adapt their design plans to ensure that the technologies they are using are delivering nothing short of the highest standard of performance.
MLCommons Releases New Results for MLPerf 2.0
On the 29th of June, MLCommons released new results for MLPerf Training Version 2.0 that sets a new performance bar. The new test results indicate up to 1.8 times better performance of training models. In this round, MLCommons focused on a new object detection benchmark. Object detection already has a broad set of use cases spanning industries – from autonomous vehicles to medical diagnosis, security, retail and much more. The reference model picked for this round has more specific uses.
We attended the pre-release briefing which was attended by people from the press and representatives from submitter companies. David Kanter, Founder and Executive Director of MLCommons and a Field Day delegate led the talk. In the first half of the session, he talked about the scope of MLPerf version 2.0 and described the RetinaNet reference model which was the chosen model for this round. This was followed by a short Q&A where participants asked questions that Kanter himself and participating companies took turn addressing.
The Scope of MLPerf Training v2.0
In each round of MLPerf, tests are performed on full systems, hardware, software and machine learning models to measure the training model performances. The v2.0 suite which is open-source and peer-reviewed like the other suites, constitutes benchmarks such as recommendation, speech recognition, NLP, 3D segmentation, reinforcement learning and image classification. This year, RetinaNet reference model was the new benchmark added to the list. RetinaNet has applications in self-driving vehicles, manufacturing and security.
Deemed as one of the most efficient ML models for one-stage object detection, RetinaNet has proven to work great with small-scale closely-packed object detection. RetinaNet surpasses other existing single-stage models of its kinds on the merit of two of its biggest improvements – focal loss function and Feature Pyramid Network (FPN). It relies on focal loss function to tackle class imbalance and compensates for the compute-intensive nature of featurized image pyramids with a less-demanding alternative. Owing to its accuracy and high speed, RetinaNet has found a place in aerial and satellite imagery.
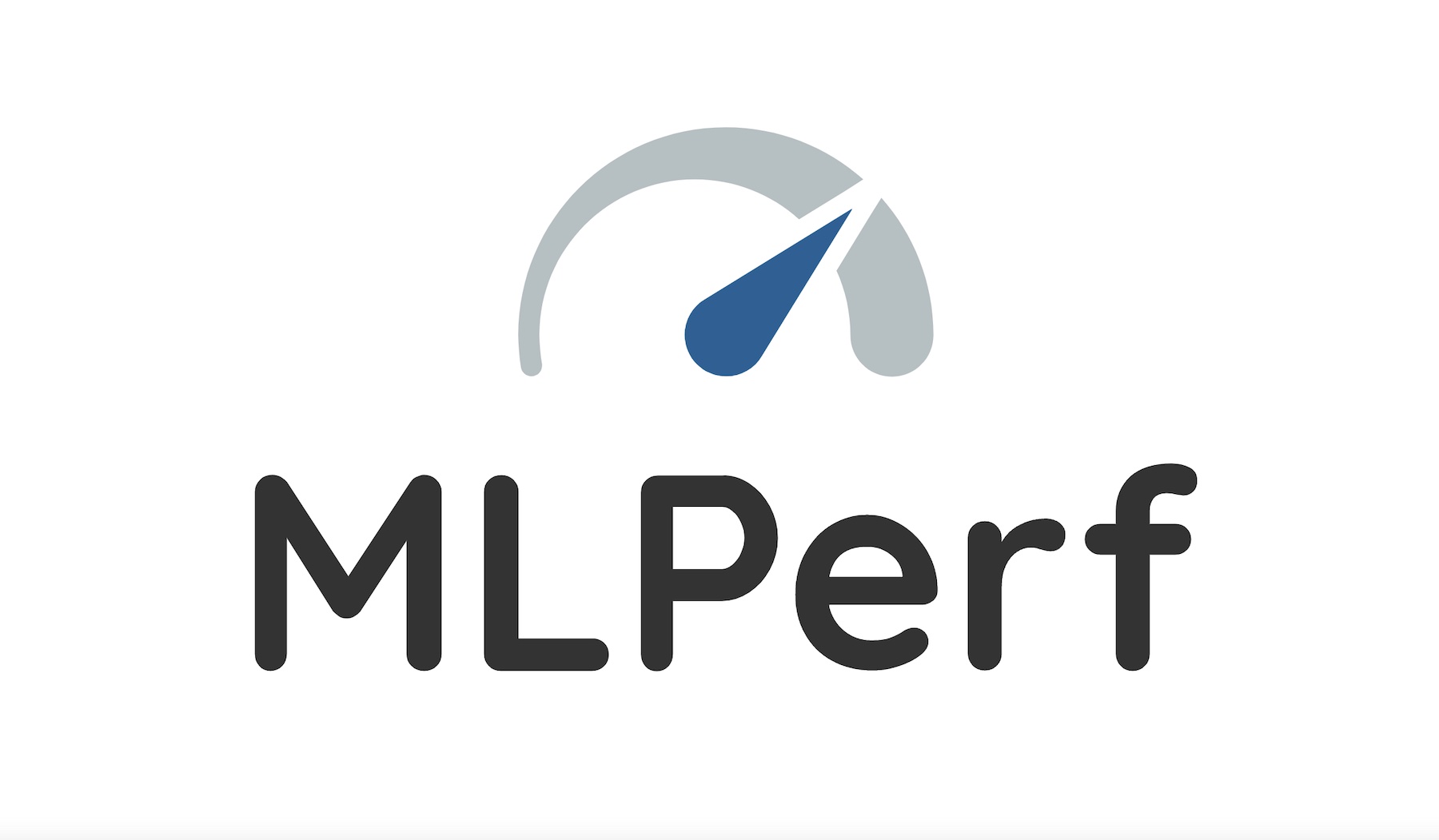
This round of MLPerf Training v2.0 received submissions from 21 companies all over the world and released over 250 peer-reviewed reports, which is significantly more results than there were in last time. There were a total of 10 different processors. It’s a positive sign that makers are coming forth in great numbers to use MLCommons’ performance exercises to demonstrate their systems’ capacity and performance for more transparency than marketing. That way, MLPerf Training v2.0 exercises are a great documentation of the evolution of AI hardware and ML models.
Participants of the MLPerf 2.0 for this round included the usual industry leaders – Azure, Dell, Baidu, Graphcore, Google, HPE and such. There were also a handful of debutant submitters that enrolled in the suite for the first time. They were ASUSTeK, Krai, MosaicML, HazyResearch, H3C and CASIA. Processors, accelerators and software in the servers submitted by each of these companies were tested to judge their performance. Systems were categorized into those available in the cloud, those that are on-prem and then there were R&D systems from Google and Netrix.
Dimensions of ML Model Training
ML models are not cheap to train, nor is it a short process. Every epoch adds big numbers to the cost of training, and you want to limit those. By adjusting the batch size, you can attain fewer epochs but it will still result in high precision. Alternately, if you decide to train your models for reasonable precision, you can go with bigger batch sizes, and for that you’ll require systems with higher throughput. So even though neither of these choices shorten the time to train or reduce computational expenses, they are the best choices enterprises have.
As for the results, each submitter brought to the table something that made their products a good fit for certain tasks. There’s no straight winner because there are too many variables involved. Making sense of the ML performance results is never easy. The system configurations are diverse, for one. For another, some systems perform exceedingly well at some tasks but fall short in others. Then there are certain systems that are overall versatile that makes great all-purpose systems but are not especially great for specific tasks. But speaking broadly, NVIDIA dominated the show in this round while Intel Habana Labs came close with pretty good numbers.
Up to 1.8 time increase in performance was noticed overall, and up to 1.49X alone at 8 processors.
However, it’s important to remember that the results are not black and white. The purpose of good benchmarking as MLCommons embodies is not to make comparisons between competitors but to share with the readers the accurate numbers based on real life applications.
In Conclusion
MLPerf Training 2.0 results are a great way to explore the options available to companies in their AI journeys. Although making sense of them may not be exactly straightforward, but going in with individual priorities and goals in mind can definitely help make the judgement of what systems make a better fit for a concerned AI project. The results, now available on MLCommons website constitute only numbers and figures, and has no marketing diversions which makes it great data to refer to before narrowing down one’s options.
Catch this week’s Rundown up top where Stephen Foskett and Tom Hollingsworth cover the MLPerf Training v2.0 results news. For more exclusives like this one, keep reading here at Gestaltit.com.