As the size of big data-driven operations like massive artificial intelligence, scientific mathematics, and financial modeling continues to grow, memory quickly becomes a limiting factor for most traditional infrastructure implementations. MemVerge displayed their answer to this issue during their AI Field Day appearance with their Big Memory Workbench solution.
Big Data and the Point of Diminishing Memory Returns
We’ve reached the point where the amount of data behind memory-driven operations like AI / ML modeling has overshadowed the ability of memory systems to contain it all. In light of this point of diminishing returns, organizations relying on traditional infrastructure environments find that they need to front extensive costs to expand their stack to meet the demands of big memory operations. On top of that, they also need to develop the necessary software infrastructure to support the expanded technology stack, eating up additional money as well as an IT department’s precious time.
Clearly, this approach is unsustainable, especially as the amount of data at play in the organization grows. And, as more AI-related use cases crop up in the enterprise, it’s apparent that companies need a memory solution that’s as big as their data.
Enter, MemVerge “Big Memory”
MemVerge built their Big Memory Workbench solution with the express purpose of addressing this need. Constructed atop their Memory Machine™ product, which, in essence, virtualizes memory across DRAM and PMEM, Big Memory Workbench consolidates memory space up to 8TB on a single server. It then allows IT personnel to interact with this big memory using either a GUI, CLI, or REST API via the various options shown in the diagram below.
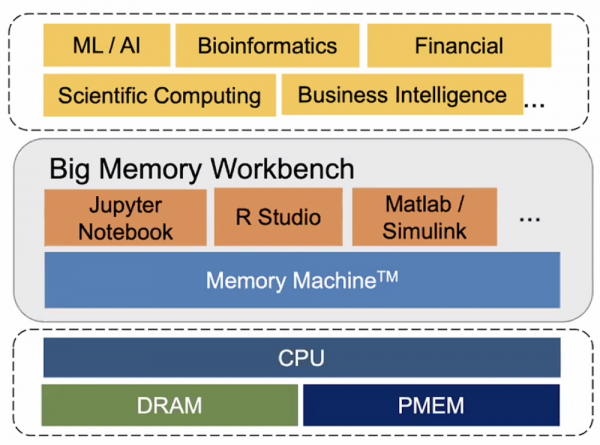
Ultimately, besides reducing costs by anywhere from 30% to 50%, thanks to software-driven memory, MemVerge’s Big Memory Workbench also significantly cuts down on the extensive I/O toll that built-out physical big memory systems inherently require. Thus, the system’s throughput remains at a higher level than it otherwise would be, creating a more ideal scenario for high-demand workflows like financial, AI / ML, and others while still providing enough memory to sustain their needs.
Security through In-Memory Snapshot Recovery
Besides the apparent benefits Big Memory Workbench provides by saving time and money for demanding workflows, it also enables IT practitioners to take routine in-memory snapshots of their workflows, which MemVerge’s Yong Tian and Yue Li detailed extensively during their AI Field Day presentation. The secret sauce behind it all is their ZeroI/O™ snapshot tool, which requires minimal overhead while still ensuring performance at scale.
By taking snapshots of memory in use, IT practitioners can leverage Big Memory Workbench to clone, migrate, and replicate their data while being used for compute processes. That way, in the case of an attempt by a bad actor, say through ransomware, IT departments can reload processes affected by the attack to avoid having to pay to recover their data; they can simply reload it themselves and get on with their day. Although the in-memory snapshots themselves add an entirely new attack vector to consider, MemVerge has built in their own security features to the Big Memory Workbench that works in tandem with your existing security tools to help ward off attacks.
Zach’s Reaction
MemVerge’s Big Memory Workbench enables IT admins to define, through software, a more efficient and effective way for expanding systems to meet the high-intensity demands of workloads like massive AI / ML or financial models, data-intensive scientific computing mathematics, and much more. Doing so not only saves organizations time and money, but also allows them to leverage more of their existing infrastructure, rather than cutting out and replacing it with more robust, expensive components.
Learn more about what MemVerge Big Memory Workbench has to offer by watching their appearance at May’s AI Field Day, or by checking out their website.