One of the biggest problems in deep neural networks is memory. There is only so much memory that a DRAM device has and DNNs frequently push DRAMs to the limit. But when you dig deeper into this memory problem, it becomes clear that neural network architectures have varying memory requirements for each stage of the data pipeline.
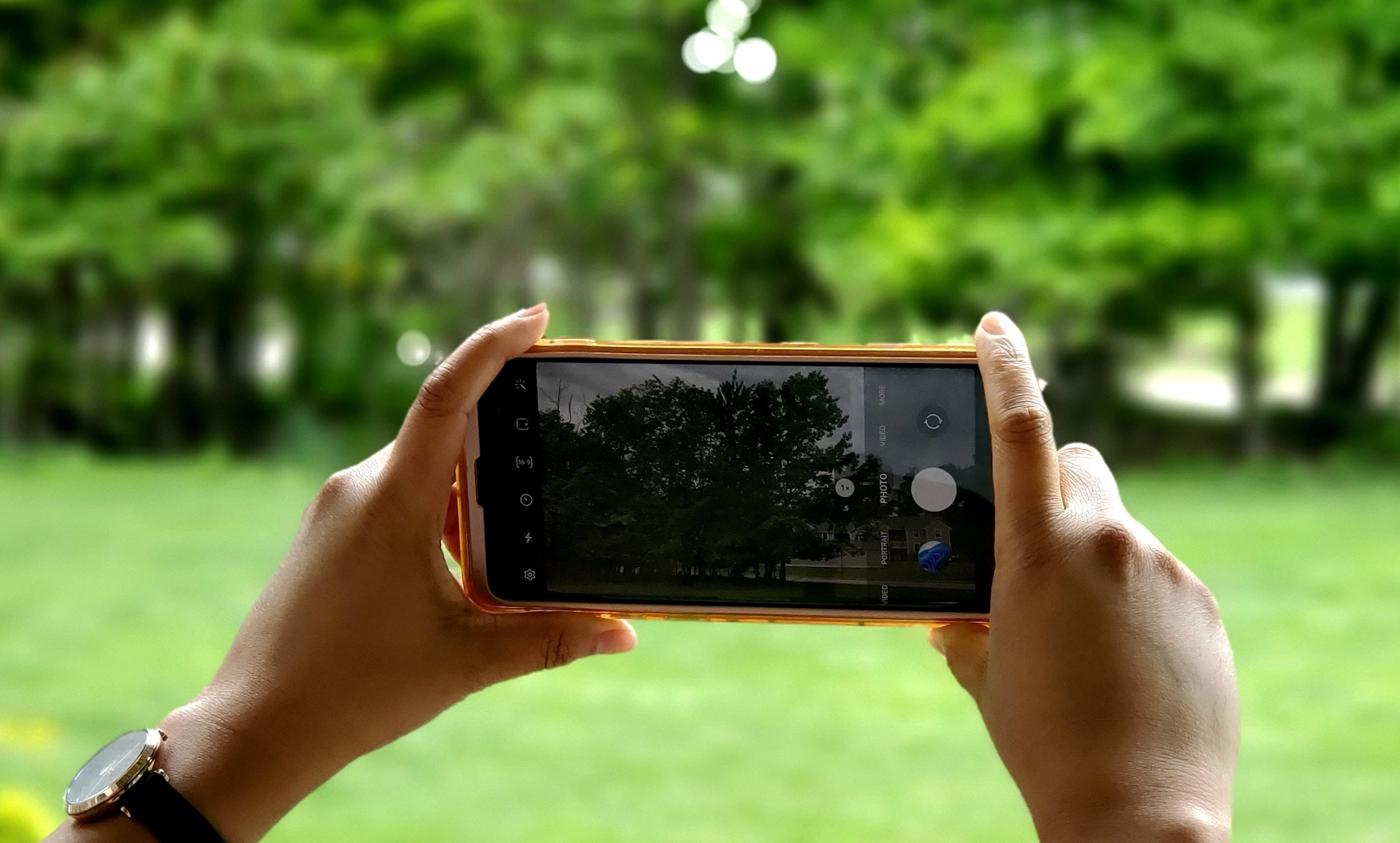
Frank Denneman, Chief Technologist at VMware has an illuminating article on this where he explains the memory consumption of neural networks at training and inferencing stages. In his article titled “TRAINING VS INFERENCE – MEMORY CONSUMPTION BY NEURAL NETWORKS” he writes,
What exactly happens when an input is presented to a neural network, and why do data scientists mainly struggle with out-of-memory errors?
Read the rest of his article -“TRAINING VS INFERENCE – MEMORY CONSUMPTION BY NEURAL NETWORKS” to find the answer to this.