Looking back at the previous two posts in this AI Storage Management series, we have defined how we would like Artificial Intelligence to help us manage our storage systems. We have also explored the data that the AI algorithms will need to make accurate suggestions and decisions. In this last post in the series, it is time to explore how the Tintri VMstore appliance and its federated management software, Tintri Global Center, leverage AI to make storage management more effortless.
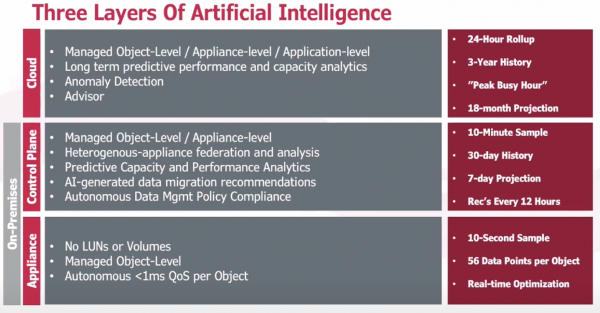
Maintaining Fair Performance with Automatic Quality of Service
Starting with day-to-day operations on a single VMstore appliance, we need to ensure that all managed objects get the performance they need, with minimal administrative intervention. On a storage system that doesn’t hit any performance limits, it’s a free-for-all. Tintri Auto-QoS is always on, though, and as soon as users hit performance limits, Auto-QoS steps in on the individual virtual machine or database level.
Auto-QoS looks back up to seven days to determine ‘normal’ behavior for a virtual machine. If the spike in I/O is abnormal and hurting other managed objects, Auto-QoS can inject latency into the I/O path to slow an object down. With this self-regulating behavior, the admin does not need to specify I/O limits manually nor adjust them later if a virtual machine grows or changes. Because it acts on an individual VM level, there is also no collateral damage like you would see when throttling an entire datastore with multiple virtual machines.
Optimizing Load Across Multiple VMstore Appliances
As your storage infrastructure grows beyond a single VMstore appliance, Tintri Global Center will become the management sphere for the collective pool of VMstore appliances. Over time, virtual machine workloads will inevitably change and could become noisy neighbors, contesting for the same resources within a VMstore.
To prevent or alleviate this load imbalance, Tintri Global Center will collect telemetry data from each VMstore once every 10 minutes. Performance metrics of managed objects are converted to TRU’s or Tintri Resource Units, enabling an honest comparison between different types of VMstores. With up to 30 days of aggregated data, TGC can find several issues and recommended actions. The algorithm looks forward to up to seven days to predict the impact that an action can have. What is left is for the admin to approve or ignore the recommended action.
A recommendation could be to move a managed object to a less busy VMstore. But the algorithms can spot recurring scheduled tasks in a virtual machine or even suggest a network or host upgrade to bring down the latency between the VMstore and the host that runs the database or virtual machine.
Organic and Planned Growth
Your virtual environment is likely to change in the future. Virtual machines or databases grow over time. Organic growth is usually relatively easy to predict with the right history. The challenge lies in the planned projects: will all these new databases and virtual machines fit?
Tintri Analytics Advisor gives you the possibility to inject planned activities such as replacements or projects into your growth estimates. It can do this based on the existing application groups that you have configured. Suppose you deploy something entirely new, though. In that case, Analytics Advisor can also leverage the combined telemetry of all VMstore appliances world-wide and give you an estimate for an average VDI desktop. With the result of a better forecast compared to guesstimating the performance and deduplication/space requirements.
These are examples of how Tintri makes storage administration more straightforward and more effective by leveraging artificial intelligence. For a closer look at the anomaly detection and the Tintri Analytics Advisor, have a look at its videos from Storage Field Day.