Among the many technologies that may shape our future, autonomous vehicles are top of the list. Love them or not, autonomous vehicles may make a durable and profound impact on how mobility is implemented, how it changes the way we travel, and how it will shape the cities of the future, hopefully for the best.
Getting there however, is a long journey with its own challenges. There are two aspects to enable autonomous travel: electronic design automation (EDA) and extensive behavior simulation through supervised reinforced learning. Let’s explore the challenges and enablers to both topics.
Live Environment Simulation
Training automated systems in real life conditions is cumbersome and expensive when you factor in the batch of hidden costs beyond the cars and hardware involved in executing these activities (labor, travel, insurance, etc.). Special authorizations may be needed at the country or state level introducing delays and complexity. And of course, unexpected accidents can also happen, with a rare but potentially lethal impact.
Simulated environments are gaining traction- they help offset most of these costs, and significantly reduce physical risks, while allowing operations to be parallelized. In addition, simulated driving conditions can be changed at will, without the need for external, real-life optimal conditions (e.g. weather, day/night, traffic density, and more).
For example, instead of feeding the algorithm with real videos of cars, images from games can be used to simulate city streets. This provides a faster time-to-value, while also allowing a faster enrichment of machine learning algorithms.
Electronic Design Automation
EDA refers to chip development, a component particularly prized in the modern automotive industry, as the recent chip shortages have demonstrated. Chip design is a time-consuming process which requires high performance environments capable of operating at scale (thousands of servers), with a powerful storage backend that can meet capacity and scalability requirements with high throughput, high IOPS and low latency, while also efficiently storing data.
In these highly concurrent environments, organizations must implement solutions that eliminate I/O bottlenecks while supporting file and object storage in a single converged solution that does not compromise on performance or scalability.
Considerations for EDA and Simulation Storage Backends
In real-life use cases, data is generated at the edge (embedded sensors) and either discarded or kept, usually for anomalous events (deviation from a standard baseline expected from a sensor, which could be anything: temperature, traffic accident, etc.) to be later sent to a core data center or the cloud. Once in the core or cloud data center, the data is processed and used for supervised reinforced learning.
However, when running in a simulated environment, the data is located either at the core data center or in public clouds. The same can be said of EDA workloads, which do not rely on data created at the edge.
The massive scale of EDA / simulation environments imposes choices to organizations: on one hand, public clouds offer outstanding flexibility especially during the growth phase. On the other hand, cloud costs can quickly spiral out of control when operated at scale.
Public clouds offer a pay-as-you-go consumption model which provides storage capabilities without the need to procure or manage infrastructure. This model is compelling for startups because of the way they are funded. They do not want to sink capital expenditure into on-premises infrastructure, but rather include infrastructure costs into operational expenses, and scale flexibly their infrastructure needs alongside business growth.
This model becomes cumbersome as the organization and its data footprint grows, but it is also impractical for incumbent players or organizations which have gone beyond the startup stage. The issues at stake are no longer about expenditure models (CapEx vs OpEx), but about intrinsic inefficiencies related to data gravity. In this case, large amounts of data are gradually stored in the cloud and on-prem repatriation becomes expensive, time-intensive, or impractical.
Another major concern is around I/O bottlenecks. Data needs to be close to compute so that it is processed as fast as possible, without any bottlenecks or latency. This requires high-capacity, high-performance storage to be adjacent to compute clusters. One last topic is around unified storage capabilities. Diverse applications and tools access data in different formats; some require file access, others object access. Without unified file and object access, additional data copy activities may even be required to make the same data sets accessible to applications using either file or object access.
Addressing EDA and Simulation Requirements with Pure Storage
To address the considerations above and meet EDA and simulation requirements, organizations could consider the advantages of using a unified fast file and object (UFFO) platform such as Pure Storage FlashBlade.
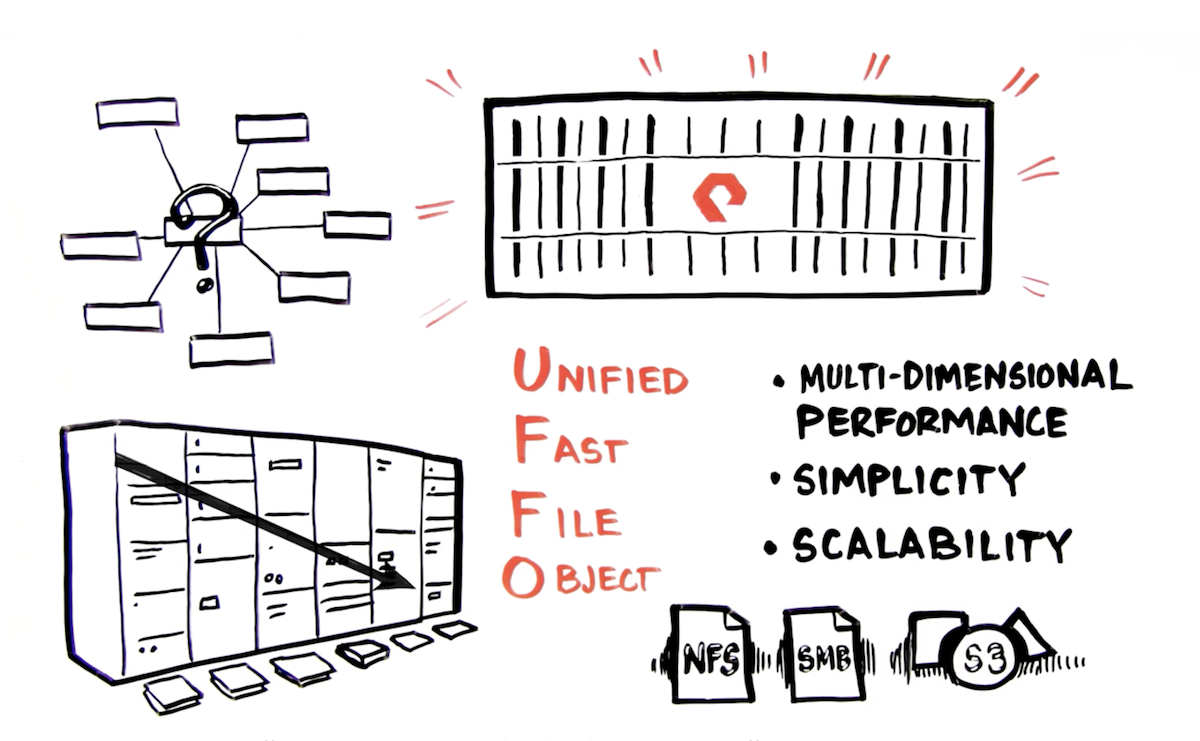
FlashBlade was purposely designed to support mission-critical research and AI/ML environments, by providing:
- Uncompromising, linearly scalable performance with petabytes of throughput and low-latency access to millions of files / objects, and exceptional performance for non-cacheable workloads
- Massive scalability with up to 150 blades per system (across multiple chassis)
- Unified file and object access, eliminating data copy operations and making data sets accessible regardless of the file protocol used, or whether the application talks file or object
- Simple and predictable storage management, thanks to Pure1 self-driving storage capabilities, drastically reducing time spent on infrastructure management and delivering valuable storage insights
- Cloud-like economics with Pure-as-a-Service, providing OpEx-based flexible consumption and reducing costs
- Seamless upgrades and expansions with Evergreen, providing peace of mind, ensuring infrastructure stability and focus on chip design and simulation / machine learning activities