The way artificial intelligence is being adopted across industries to consummate myriads of use cases have a profound impact on the way things have been done through generations.
Planning a Surprise Birthday Party to Performing Hard Math
Since the unveiling of AI chatbots, people have been tinkering with them frequently, finding creative ways to leverage their natural language processing skills. Some examples would be using them as personal shoppers to avoid extensive product research, turning them into life coaches to help shape and organize goals and milestones, getting them to produce an instant poem, or a recipe, using them as study pals before exams, so on and so forth.
Turns out, artificial intelligence is pretty good at these daily tasks. But there are industries for which AI’s logic and reasoning yields far greater payoffs. In medicine for instance, assistive technology has proven key to helping patients manage symptoms of diabetes or overcome handicap of blindness. In finance, it is helping boost profits to new highs through high-frequency analysis and fraud detection.
“The capabilities and use cases of AI are vast and wide, and as such will be deployed by many enterprises,” said Nemanja Kamenica, Technical Marketing Engineer at Data Center Networking Group at Cisco, at the recent Tech Field Day Extra at Cisco Live US 2023 event.
In a presentation, Mr. Kamenica showcased Cisco’s Data Center Networking Blueprint for AI/ML Applications, a handbook that provides an industry-approved network model for AI/ML workloads.
Under the Hood of AI Models
AI models live and die by the network. The cutting-edge workloads fare best in high-performance AI/ML networks that have resources to support and optimize the performance of data-driven algorithms. So, they demand low-latency, lossless networks to operate at full efficacy.
How does it all work? Enterprises’ AI/ML initiatives begin with putting together a colossal repository of data scraped off of countless sources. This data goes into training the AI models to perform an endless variety of complex tasks. The more training data you bring in, the better results the algorithms produce.
At the ground level, all AI/ML workloads have the same objective – to produce output against datapoints or a sequence of text. Whether the ML models produce a perfect match or one that is close to an acceptable match depends largely on how well the models function.
Mr. Kamenica touched upon two types of AI clusters and the way they interact with the network. The first is distributed training cluster where the training happens. The distributed training cluster relies on node to node transfer of data for which it requires high node to node bandwidth, and low latency transport. To ensure a short training time, this cluster needs to have a lot of CPU and GPU firepower on standby. So a large network with numerous CPU or GPU hosts is ideal.
The second type is production inference cluster where the models are trained to infer. The first requisite of this workload is high availability and low latency, so that anytime a user asks a question, they get a prompt response. This requires smaller networks with mid-size CPU/GPU hosts.
Building out an AI/ML Network
Whether an enterprise chooses to build their AI cluster on-premises or in the public cloud, there is a set of challenges they face. First is the matter of scalability. AI models are data hungry. Data doubles every two months, which means the infrastructure needs to grow at the same scale to keep up. At the minimum, a cluster should have 512 GPUs. The larger the size, the shorter the training time.
So the way to build this network is to use hardware and software that have the capabilities to provide the bandwidth, latency and congestion management features required for AI/ML applications. Cisco’s Nexus 9000 Series checks these requirements. The Nexus 9000 Series switches help build a network that offers high throughout and low latency with congestion management capabilities that allow few to no traffic drops.
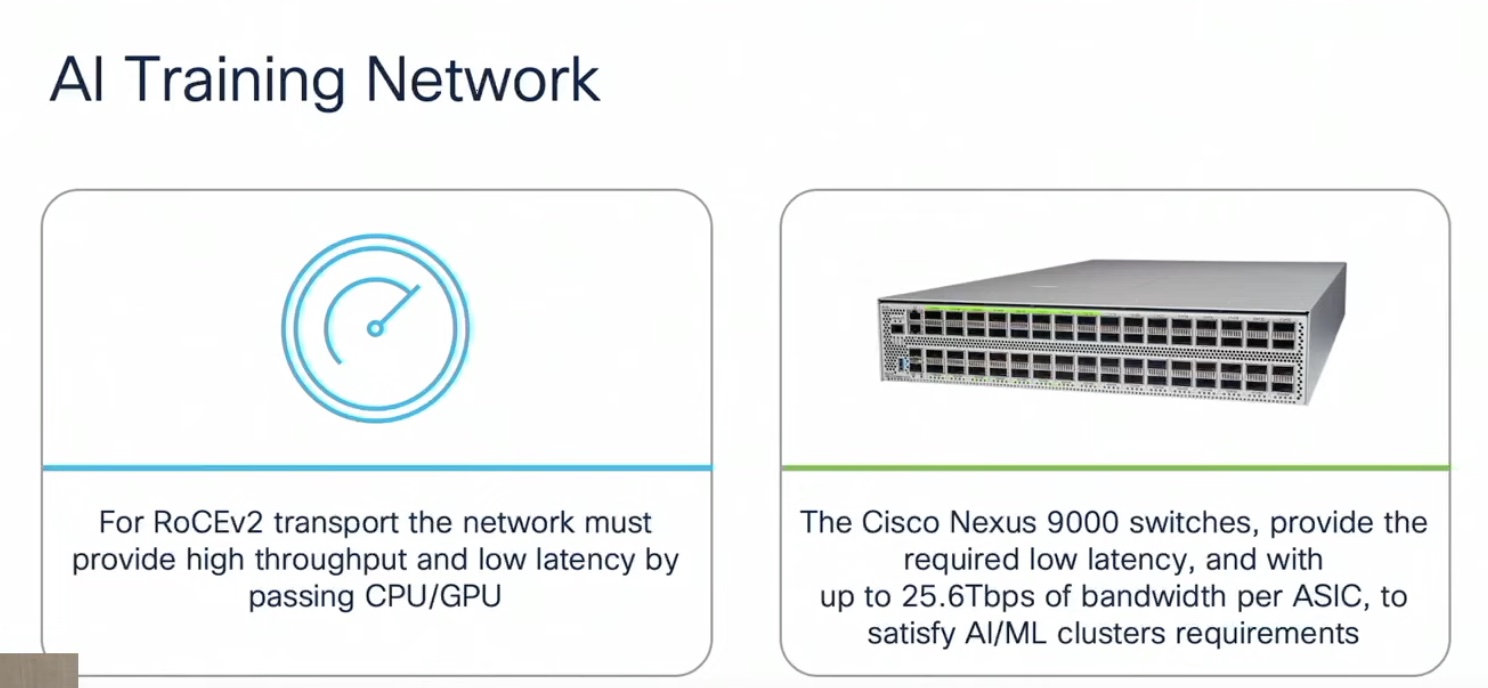
A preferred technology for high throughput low-latency transfer is RDMA or Remote Direct Memory Access. RDMA allows fast transfer of information between nodes without overworking the CPU or consuming extra power.
One of the implementations for network transport it provides is RDMA over Converged Ethernet, also referred to as RoCE. The second version of this protocol, RoCE v2 came in 2014, and found wide adoption in datacenter networks because of the ubiquity of ethernet in datacenters.
While RocE v2 is an excellent transport, it requires a lossless network to deliver its benefits. This is where Cisco’s hardware comes in.
The Cisco Nexus 9000 datacenter portfolio comprises of switches that offer speed from 100Mbps to up to 400Gbps. Running on two operating systems in standalone and ACI modes, these appliances are based on Cisco silicon which enables a set of common features by default. The switches provide bandwidth of up to 25.6Tbps per ASIC which is at par with what’s required for AI/ML clusters.
Additionally, the Nexus 9000 software tools like the Nexus Dashboard Insights and Fabric Controller complete the platform with advanced automation capabilities for AI/ML applications. The dashboard facilitates network management by offering visibility through telemetry, telling operators which GPUs are suitable for certain processes, and help allocate resources granularly based on the requirement.
Wrapping up
Enterprises will not be able to make good on the promises of artificial intelligence without a network that can transport massive amounts of global data reliably at flashing fast speed. The Cisco Data Center Networking Blueprint for AI/ML applications provides a reference architecture for achieving that reliability and connectivity to support this new wave of innovation at highest efficiency and lowest friction. If the goal is to join the AI/ML bandwagon, it is never too early to start planning.
Grab a copy of the document today or watch the full presentation from the recent Tech Field Day Extra at Cisco Live US 2023 event to understand the strict infrastructure requirements of AI/ML clusters and the best practices to meet them.