There is nary a trend today that looms as large as artificial intelligence, and everybody wants a slice. New hardware products leap out of the assembly lines like clockwork, vitalizing this revolution. In particular, GPUs capture a large mindshare and market share for its superior performance. Packed with infinite compute power and sub-millisecond latency, they have emerged as the hottest commodity in the hardware market.
But as chip shortage and long wait-times leave companies hungry and scrambling for compute power, on the inference side where the AI algorithms make sense of the data, CPUs are making a comeback.
Intel has been readying an alternative to seize this opportunity for a while. After Habana Labs Gaudi, the 5th Generation Intel Xeon Scalable Processor – nicknamed Emerald Rapids – is the new prize. Xeon processors, with adequate performance and millisecond latency, are poised to set off a wave in general-purpose AI market.
At the recent AI Field Day event in California, hosted by Tech Field Day, part of The Futurum Group, where Intel hosted a full-day presentation (perfectly summarized here by Paul Nashawaty, Futurum Group analyst), partners and customers like VMware by Broadcom, Google Cloud, Kamiwaza and Nature Fresh Farms, shared their success stories of deploying CPUs for private AI.
In back-to-back sessions, Ro Shah, AI Product Director at Intel, outlines how the growing use of CPUs in AI inferencing is changing the picture, and elaborates it through examples of Intel partners.
A Lighter Alternative for Lean Models
So what’s causing enterprises to shift from the big beefy GPUs to these skinner cousins? AI models generally fall into one of two camps – massive models that have billions and trillions of parameters, and the lighter, nimble models that are about billion parameters strong.
“That’s where we’re expecting to see a majority of enterprises to end up because of the cost associated with deploying a trillion plus parameter model, and the ability to leverage capabilities like retrieval augmented generation (RAG), and fine-tune models to specific use cases,” says Shah.
A wide variety of models fit into this latter category. As more enterprises pivot to private AI, the general-purpose AI bucket is filling up fast. For mixed workloads, where AI is one of many, CPUs are increasing becoming the hardware of choice.
Shah explains that general purpose AI comprises applications like real-time audio-video, group chat, screen sharing, recording and such. These workloads have a relatively low set of throughput and latency requirements that are more akin to what CPUs offer.
“A lot of it comes down to how fast we can run those AI cycles on CPUs,” says Shah.
Xeon fits right into this market. Intel has prepped the Xeon brand of CPUs with the right kind of power and performance that can knock these requirements out of the park without trouble. Loaded with the trademark Advanced Matrix Extensions (AMX), the cores deliver max performance for deep-learning inference.
Not a Replacement for GPUs
But Intel is not marketing Xeon as a contender to GPUs. Right out the gate, Shah makes it plain that the Intel Sapphire Rapids are not a replacement for GPUs in bigger models. “If you’re inferencing a trillion-parameter model like GPT-4 where a majority of the cycles are AI cycles, it makes sense to go for an accelerator,” he states.
Some of the biggest reasons for deploying CPUs for AI inferencing are the relatively lower latency requirements of general-purpose AI workloads, cringy costs of GPUs, and deployment complexities.
Intel’s Xeon CPUs sustainably meet the critical requirements of multi-workloads, while trimming down cost and complexity.
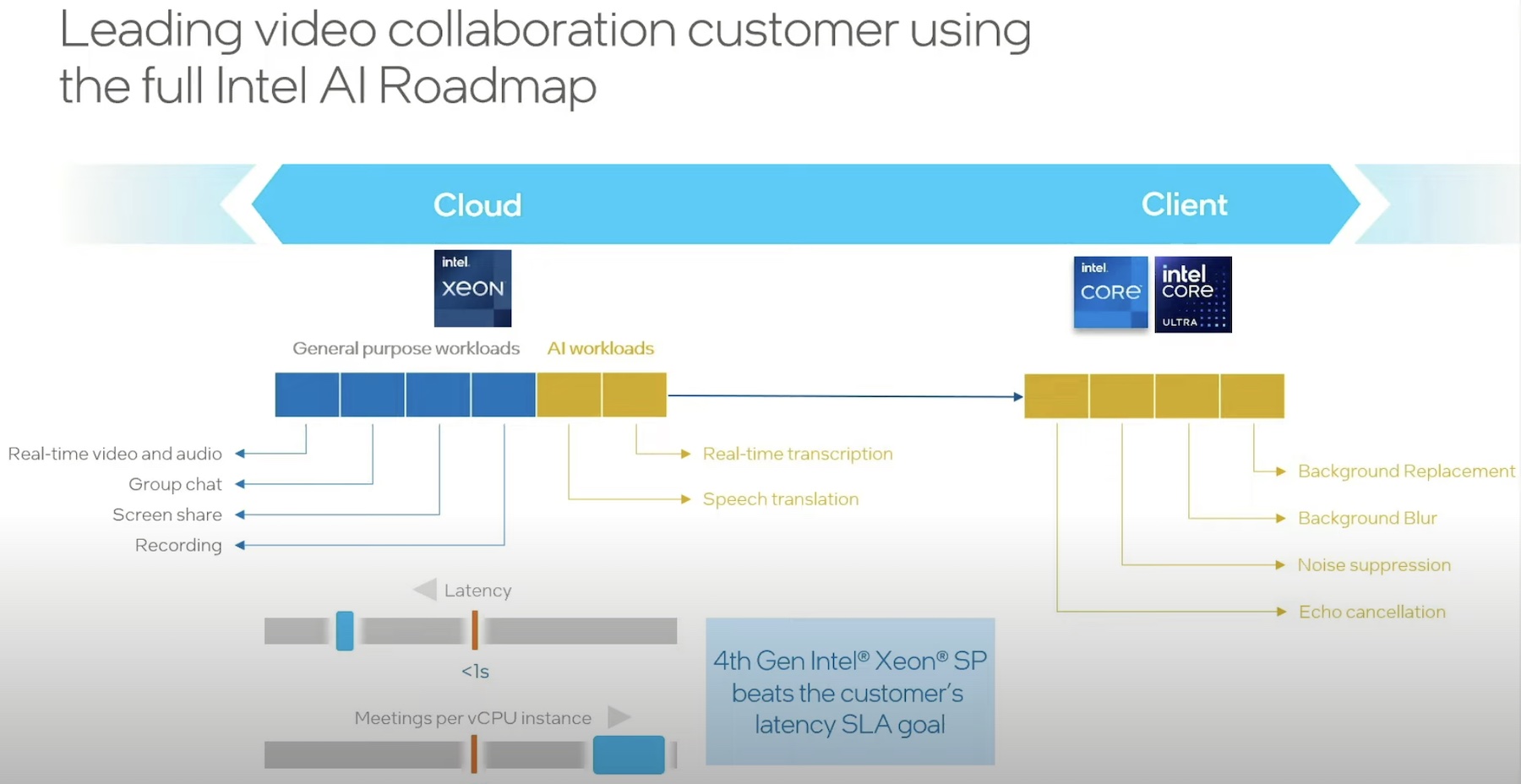
Real-World Numbers
Enterprises working with models south of 20 billion parameters has a baseline requirement of 100 millisecond latency. Shah gave the example of a couple well-known models to establish this. GPT-J, a 6 billion parameter model, has next token latency requirement of 100 ms. In plain-speak, next token latency is the speed at which a model can render a full response. At a 100 milliseconds rate, it safely surpasses the average adult reading speed, which is what the goal is.
For a model of its size, the 5th Gen Xeon Scalable Processor provides a 30 ms latency, which, for a relatively larger model like Llama 2 that has13B parameter, is 60 ms – still well within the threshold value.
Of course, to realize these numbers, all cores have to be engaged at once. However, if the workload can do with less, fewer cores can be put to use to push up the latency and increase the number of concurrent users, says Shah.
Shah shares datapoints from case studies to demonstrate Xeon’s performance and throughput benchmarks in AI inferencing. For a certain customer doing Resnet-50 batch inferencing, Emerald Rapids delivered a whopping 19,000 frames per second.
“If you’re supporting cameras, that’s hundreds of cameras you can support,” says Shah.
It’s important to note that high outcomes can be achieved without utilizing all cores. “You don’t need to use the entire CPU socket to deploy your AI inference. You only need a few cores to do your AI, and you can use the rest of the cores for your general-purpose application,” says Shah. This unlocks significant TCO benefits which is one of the top priorities of smaller companies.
“This is not to say that in every scenario less than 20 billion, you should use a CPU, but 20 billion is where we can meet your critical requirements,” he reminds.
Intel pledges even higher performance and lower latency in the future generations of Xeon making it possible to deploy bigger deep-learning models on fewer CPU cores.
Wrapping Up
As artificial intelligence becomes ubiquitous with more companies incorporating AI workloads, a gap has opened up dividing enterprises engaged in big-money projects from the smaller guys that are dabbling in general-purpose AI. Intel has cleverly tapped into this, bringing to a class of underserved customers a product that meets them in the middle, and affords them the chance to stay competitive. Xeon neither takes big spending power, nor comes with long wait-lists. Instead, it alleviates the anxiety around scarce access to GPUs by showing that AI can be kicked off perfectly with less powerful hardware.
Watch Shah’s full presentation to know how Intel is continuing the work on the software level to accelerate AI. Don’t forget to check out the presentations from VMware by Broadcom, Google Cloud, Kamiwaza, and Nature Fresh Farms, from AI Field Day event that elaborate on the suitability of CPUs for AI inferencing.